
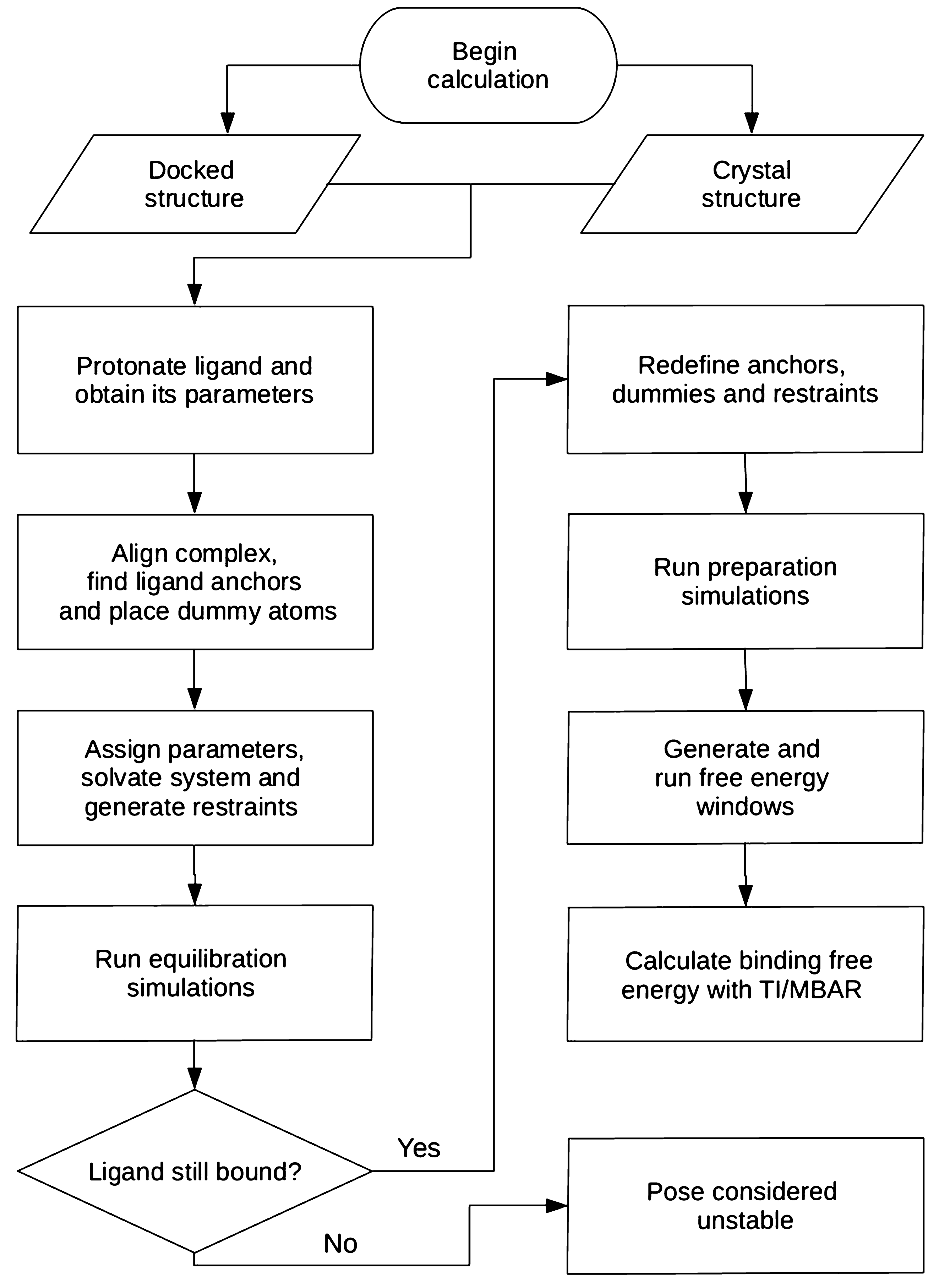
QSAR approaches are not restricted to cases where experimentally determined structures are available they can be applied purely from structure and activity data.
#Gaussian software to know charge of binding pocket free
For single perturbations of a few atoms, errors in predicting changes in free energy were approximately 0.9 kcal/mol. Recent advances in force-fields, sampling methods, and automated design of perturbation graphs have yielded results that offer guidance in fine-grained molecular optimization. For the FEP approach, affinity predictions are made by estimating the difference in the free energy of protein-ligand complexes between closely-related ligand pairs (typically just a few substituent atoms are different). For the former methods, in the case where experimental structures are known for all ligands under consideration, performance can be quite variable based on the specific protein target (Pearson’s r \(^2\) range of 0.0–0.8 for individual proteins), though careful work has shown more consistent results in some cases (r \(^2\) of 0.5–0.6 for three enzyme targets). These methods vary in the degree to which they parallel what is known physically about protein-ligand interactions.Ī key attraction of simulation oriented physics-based methods, such as MM/PBSA or MM/GBSA or free-energy perturbation (FEP), is that, in principle, these approaches are congruent with what we know physically. Catalyst and Phase), and descriptor-based approaches. The most widely used methods for activity prediction in such cases include field-based approaches such as CoMFA (and variants such as CoMSIA, Topomer CoMFA, and CMF), pharmacophoric approaches (e.g. Experimental structure determination remains challenging for many pharmaceutical targets such as ligand-gated ion channels, membrane transporters, and (now to a lesser extent) membrane-spanning G-protein coupled receptors.
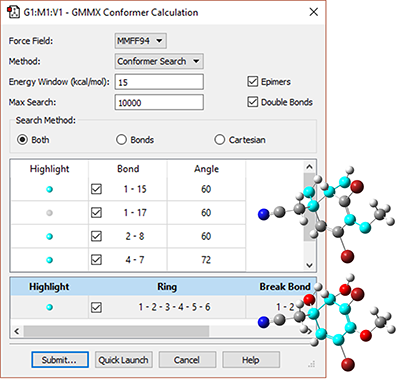
The method is applicable for fine-grained lead optimization as well as potent new lead identification.īinding affinity prediction continues to be a challenge for computer-aided drug design, especially in the case where there is no high-resolution experimental structure of the target of interest.
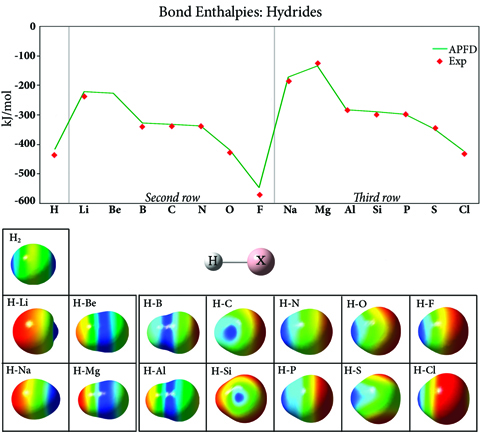
QuanSA predictions yield binding affinities, explicit estimates of ligand strain, associated ligand pose families, and estimates of structural novelty and confidence. Further, we show that combining predictions from QuanSA models with those from physics-based simulation approaches is synergistic. In particular, we show how models initially induced from small data sets can extrapolatively identify potent new ligands with novel underlying scaffolds with very high specificity. We provide algorithmic details along with performance results on sixteen structure-activity data sets covering many pharmaceutically relevant targets. The problem of mutual ligand alignment is addressed in a general way, and optimal model parameters and ligand poses are identified through multiple-instance machine learning. The method is closely related to the QMOD approach, substituting a learned scoring field for a pocket constructed of molecular fragments.
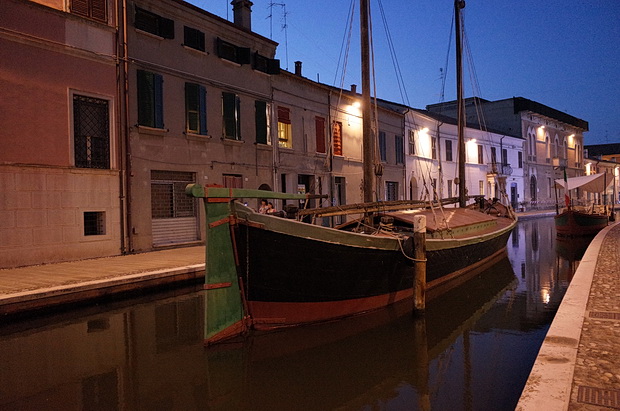
We introduce the QuanSA method for inducing physically meaningful field-based models of ligand binding pockets based on structure-activity data alone.
